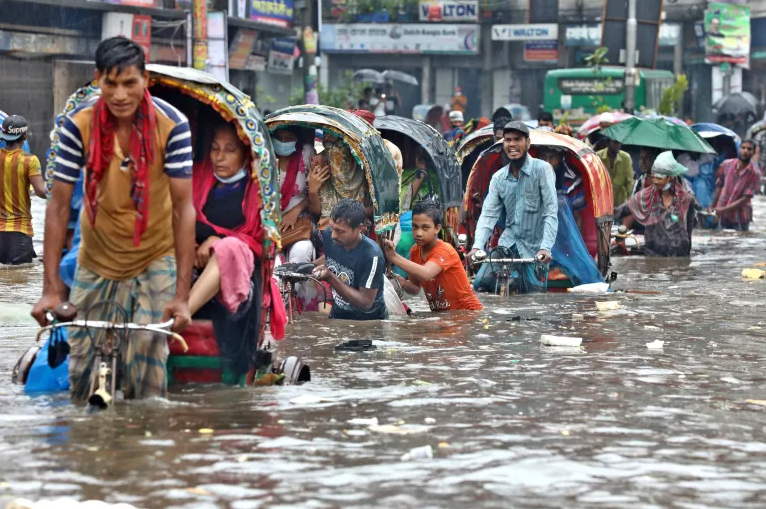
In the past five years, a new generation of weather prediction models has emerged, categorized into two types: hybrid models that integrate old models with artificial intelligence (AI) systems, and models entirely based on AI systems. Major tech companies have been behind the development of the latter type.
An example of a hybrid model is the HCCA (Hurricane Center Composite Approach) system used by the U.S. hurricane center. This new HCCA system integrates inputs from multiple models and uses machine learning to rely more on models that have performed better based on past forecasts. An example is Hurricane Ida in 2023, where the average path error for HCCA over a three-day period was 97 nautical miles, compared to 116 for official forecasts.
Both types represent meaningful improvements, as the cost of evacuation is approximately a million dollars per mile. Additionally, the model outperforms official track forecasts for several storms in 2023. Models relying entirely on artificial intelligence are even more impressive, and understanding how they operate is crucial.
AI models are trained on massive amounts of historical data, and algorithms analyze this data to find relationships between previous observations or forecasts and subsequent conditions. Using machine learning algorithms, AI systems can analyze all this information in a fraction of the time and with greater accuracy and efficiency than traditional models, avoiding the risk of human error. By examining historical weather patterns and comparing them with current conditions, AI models can predict potential anomalous situations that may lead to natural disasters.
Once trained, AI models can generate predictions in seconds to a few minutes on any available computer, compared to over an hour on large supercomputers for most traditional models. While the concept is similar to how meteorologists use their expertise to predict weather deviations from model expectations, AI does it on a scale and speed that is challenging for humans to match.
Leave a Reply