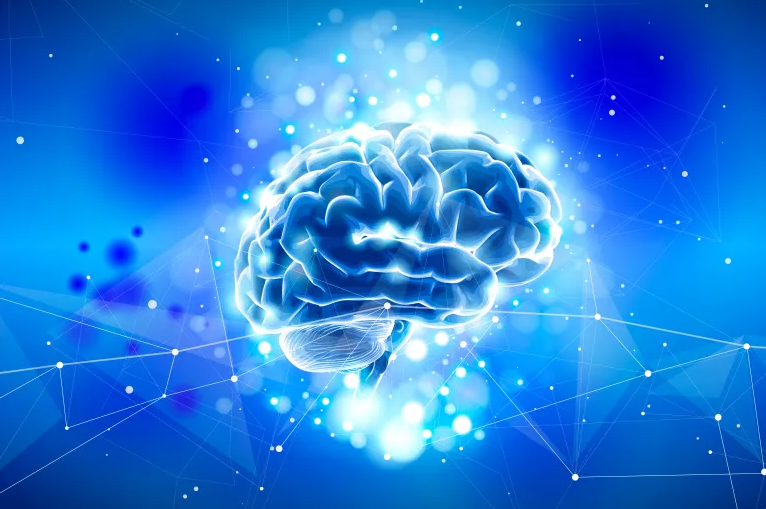
A recent study from the University of Oxford suggests that the human brain relies on fundamentally different and more efficient learning and cognitive acquisition processes compared to current artificial intelligence (AI) systems and mechanisms. This challenges the prevailing belief that the learning capability of artificial intelligence surpasses that of humans.
Most contemporary artificial intelligence systems, including those incorporating artificial neural networks resembling the human brain, use a process called backpropagation. This process involves teaching artificial neural networks by backpropagating information in the opposite direction to the original flow, adjusting weights and connections between neurons in case of errors. This fine-tunes the decision-making process to achieve the correct answer.
In contrast, the human brain is capable of learning from a single instance, while artificial intelligence requires hundreds of repetitions of the same information to achieve accurate results. Additionally, human learning does not necessitate a pre-existing database, unlike artificial intelligence.
The study, titled “Pre-formation neural activity as a basis for learning away from backpropagation algorithms,” indicates that the human brain outperforms artificial intelligence in various ways. While artificial intelligence may excel in specific tasks, it requires hundreds or thousands of training iterations to learn, unlike humans who can learn from a single experience regardless of existing knowledge.
The researchers propose an alternative learning method called “future shaping.” Instead of modifying neural connections, the activity of neural cells changes to better predict outcomes, followed by adjustments to weights and connections to match the new pattern.
Computer simulations have shown that models using future shaping can learn more efficiently than traditional neural networks in artificial intelligence, especially for tasks related to living organisms.
Despite its clear advantages, implementing future shaping technology on existing computers poses a challenge due to their fundamental differences from the biological brain. The first author of the study, Dr. Yuhang Song, emphasizes the need to develop new types of computers or brain-inspired devices to use this approach with minimal energy consumption.
The lead researcher, Dr. Rafal Bogacz, highlights the current knowledge gap between theoretical models of future shaping and understanding the anatomy of the human brain network. He underscores the importance of future research addressing this gap and exploring the potential of the most efficient and beneficial learning method.
Leave a Reply